The Future of Creativity Exploring the Power of Generative AI
Generative AI refers to a class of artificial intelligence techniques designed to create new, original content by learning from existing data.
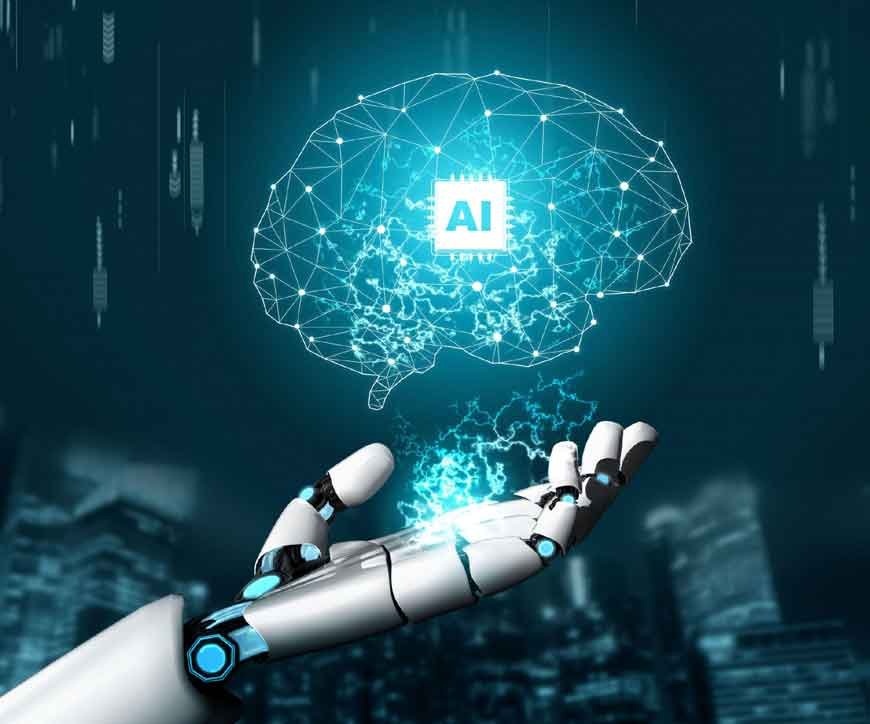
Imagine a world in which machines not only help humans with our creative activities, but also become artists, authors, and musicians themselves. Welcome to the world of generative AI, a fascinating mix of technology and creativity that is changing the way we approach art, design, and problem solving. From dazzling images generated by neural networks to fascinating narratives created through natural language processing, generative models are altering the landscape of creative expression.
As deep learning techniques advance, so does their potential to drive innovation across multiple industries. Whether it's using style transfer to create one-of-a-kind artworks or reinforcement learning for interactive storytelling, generative AI offers up new possibilities that were previously only available to the human imagination.
Curious about what motivates this revolution?
Let's look at the key technologies driving generative AI and see how they're changing not only how we create, but also who gets to be deemed a creator.
Key technologies behind generative AI
Generative AI relies on a number of important technologies to provide its extraordinary powers. Deep Learning techniques are key to this revolution because they enable robots to learn from large datasets and simulate human-like inventiveness.
Among these, Generative Adversarial Networks (GANs) are notable for their distinct architecture. They are made up of two neural networks: a generator that produces material and a discriminator that assesses its authenticity. This dynamic drives both networks to continue improve.
Variational Autoencoders (VAEs) play an important role in generating new data points from old ones. They efficiently capture complicated patterns in data, making them excellent for applications such as image synthesis.
Natural Language Processing (NLP) improves text-based creativity by enabling robots to construct cohesive stories or poems. Techniques like Style Transfer enhance creative productivity by effortlessly integrating multiple artistic styles into new shapes. Together, these tools open up a new frontier for generative AI research.
How Does Generative AI Work?
Generative AI uses complex algorithms to create fresh material. At its heart, it examines large datasets to discover patterns and structures within them.
Deep learning is critical in this context, as it employs neural networks capable of mimicking human-like creativity. These networks learn from prior examples, allowing them to produce unique results based on learnt patterns.
One common technique is Generative Adversarial Networks (GANs). In this configuration, two models—the generator and the discriminator—compete against one another. The generator creates samples, and the discriminator assesses their legitimacy.
Another approach uses Variational Autoencoders (VAEs). They compress input data before reconstructing it, allowing for differences in output while preserving fundamental aspects of the original data. These methods enable everything from text-to-image generation to music composition, demonstrating how generative AI broadens creative potential across multiple disciplines.
Applications of Generative AI.
Generative AI is transforming numerous industries through its novel applications. One of the most exciting areas is that of art and design. Artists are employing AI-generated art to push creative boundaries by combining human intuition and machine learning.
In the entertainment industry, generative models create one-of-a-kind music and film scripts. This confluence produces innovative narrative strategies that fascinate viewers.
In marketing, firms use text-to-image creation systems to quickly create targeted adverts. This not only increases engagement but also improves production efficiency.
Generative Adversarial Networks (GANs) also benefit healthcare by providing advanced medical imaging techniques that improve diagnosis and treatment regimens.
Educational systems leverage creative AI to create interesting information that is suited to individual learning styles. By evaluating data trends, these systems dynamically adapt classes to improve student retention rates.
Benefits of Generative AI
Generative AI has numerous benefits across multiple industries. One major advantage is its capacity to boost creativity. Artists, designers, and writers can use these resources to spark their imaginations or overcome creative blockages.
Another advantage is efficiency. Generative models can automate time-consuming processes, freeing creators to concentrate on more strategic parts of their work. This allows for speedier project turnaround times without losing quality.
Furthermore, generative AI allows for unparalleled levels of customisation. Businesses can generate individualized content that appeals to specific users, increasing engagement and happiness.
Cost-effectiveness is also important. Organizations save money by streamlining procedures and lowering the need for substantial human resources while delivering high-quality results.
Additionally, it promotes experimentation. Advanced techniques such as style transfer and data augmentation enable authors to experiment with novel ideas without fear of failure.
Ethical Considerations for Generative AI
Ethical considerations in generative AI are crucial as the technology advances. The potential to create AI-generated art and prose poses issues of ownership and authorship.
Who owns the work done by machines?
This ambiguity can lead to disagreements.
Furthermore, there is fear about misinformation. Generative algorithms can generate realistic fake news pieces or deepfakes, confounding our grasp of the truth in media.
Privacy concerns also arise. Using personal data to train these algorithms involves dangers that must be carefully managed.
Another important aspect is bias in neural networks. If the data given into these algorithms is biased, the results will reflect those prejudices, thereby propagating stereotypes.
Accountability is a grey area. As machines develop material on their own, assigning blame for bad outcomes becomes more difficult, sparking critical debates about regulation and ethical principles in creative AI applications.
Limitations and Challenges
Generative AI, while its potential, has many limitations and obstacles. The quality of the output is an important consideration. While models such as Generative Adversarial Networks (GANs) can generate visually appealing images or text, they frequently struggle with coherence and context.
Data bias is also a big concern. If the training data reflects societal biases, the resulting content will most likely reinforce these problems. This could result in ignorance or negative stereotypes.
Additionally, computational expenditures are significant. Deep learning models demand a significant amount of time and energy to train. Not every organization has access to the required technology or money.
Intellectual property rights present another problem in this changing context. As AI creates art or music, problems of ownership and attribution arise. Navigating these legal waters is challenging for both creators and businesses.
Ethical considerations cannot be neglected. How can we make sure that generative models are used responsibly? As this sector advances quickly, it is critical to strike a balance between innovation and caution.
The Future Of Generative AI
The future of generative AI is filled with possibilities. As technology advances, we may see even more complex uses emerge. Consider bespoke information suited to individual preferences, created seamlessly by algorithms.
Advances in deep learning will push the boundaries even further. The use of Generative Adversarial Networks (GANs) and Variational Autoencoders (VAEs) is only the beginning. They open the path for developments that blur the distinction between human creativity and artificial intelligence.
Text-to-image generation has the potential to transform the way we visualize concepts. Artists may collaborate with AI to create amazing images or immersive experiences that improve narrative across several mediums.
Natural Language Processing improves human-machine communication. This could result in smarter chatbots or interactive narratives that adjust based on user feedback.
As generative models become more available through AutoML-powered platforms, a new wave of creators will emerge, using these tools for artistic expression in ways we can't yet envision.
Industry Impact of Generative AI
Generative AI is altering industries around the world. It has an undeniable influence on everything from art to healthcare. Businesses are using generative models to develop creative solutions and streamline procedures.
Artists in the creative sector use AI-generated art to inspire and collaborate. This confluence of technology and creativity produces new artistic expressions that push established boundaries.
Deep learning techniques, such as Generative Adversarial Networks (GANs), provide considerable benefits to the gaming industry. These let developers to create realistic surroundings and characters, which improves player experiences.
Healthcare sees dramatic potential in data augmentation. Medical imaging can benefit from synthetic data created by neural networks, which allows for better training of diagnostic tools.
Furthermore, corporations use natural language processing in customer service applications. Generative AI-powered chatbots deliver tailored interactions on a large scale, increasing customer pleasure while decreasing operating expenses.
Applications of Generative AI.
Generative AI is transforming industries by enabling previously inconceivable uses. In the creative arts, artists use models like Generative Adversarial Networks (GANs) to create breathtaking graphics and one-of-a-kind artworks.
In the fashion industry, designers use deep learning algorithms to create new garment patterns and trends. This not only speeds up the design process, but it also improves collection uniqueness.
The game sector also benefits from generative models. Text-to-image technology allows developers to create huge virtual worlds with different sceneries. This innovation promotes immersive experiences for players.
In addition, firms are using natural language processing systems to create targeted marketing content or generate reports quickly. These improvements improve operations while preserving creativity and engagement.
Healthcare is another industry undergoing upheaval due to data augmentation enabled by generative AI, which provides fresh insights into patient care and treatment alternatives without jeopardizing privacy or ethical norms.
Key techniques in generative AI
Generative AI uses a variety of important strategies to generate material that mimics human ingenuity. One of the most well-known methods is Generative Adversarial Networks (GANs). They involve two neural networks, the generator and the discriminator, competing with each other to improve output quality over time.
Variational Autoencoders (VAEs) are another useful tool for learning efficient data representations. VAEs are especially useful for creating images or complex structures by collecting underlying patterns.
Text-to-image generation has recently acquired popularity. This approach allows users to enter descriptive language, which is then translated into graphics using deep learning algorithms.
Natural Language Processing is also important in this arena, as it allows machines to interpret and generate text that is similar to human language. These strategies work smoothly alongside others such as Style Transfer and Reinforcement Learning, boosting creative ventures across industries and pushing boundaries further than ever before.
Key Concepts of Generative AI
Generative AI exists at the crossroads of creativity and technology, altering our perception of what is possible in art, design, and even writing. At its core is a commitment to innovation using generative models that learn from large datasets. These systems can generate novel material by utilizing deep learning techniques such as Generative Adversarial Networks (GANs) and Variational Autoencoders (VAEs), which frequently blurs the boundary between man-made and machine-generated.
This powerful technology's foundation is built on core principles such as neural networks. They allow machines to identify patterns in data, opening the door for advances in Natural Language Processing and Text-to-Image Generation. This enables creatives to create artwork or prose with simple suggestions, transforming ideas into visual masterpieces or coherent tales with astonishing ease.
Furthermore, techniques such as style transfer demonstrate how Generative AI may imbue new products with artistic flair evocative of established styles. The potential applications are boundless; from improving data augmentation procedures to optimizing workflows, AutoML (Automated Machine Learning) empowers people across industries.
As we go deeper into this arena, ethical questions must stay front and center. Questions around ownership rights for AI-produced art, as well as worries about misinformation generated by advanced language models, necessitate critical social debate.
Generative AI is more than a buzzword; it is a disruptive force that is redefining creativity while also bringing exciting opportunities and challenging obstacles. Embracing these fundamental notions will be critical as we navigate an era in which human creativity works seamlessly with artificial intelligence—a partnership replete with untapped potential poised to influence our future landscape.
What's Your Reaction?
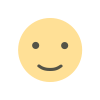
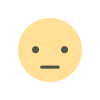
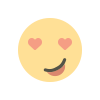
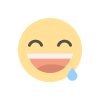
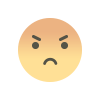
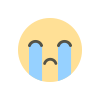
